DATA QUALITY & GOVERNANCE
Transforming data into actionable information and applied knowledge to empower your business
5 minutes read
What is Data Quality & Data Governance?
Data quality and data governance are essential for every modern business that wishes to be a successful data-driven enterprise.
Typically, organizations acquire data quality solutions to “cleanse” their data in the belief that their data problems would be resolved permanently. In every instance, it does not operate that way.
What is Data Quality?
This refers to the ongoing practice of ensuring that data is correct, comprehensive, and consistent in order to successfully manage an influential commercial organization.
(To put it another way: it’s the current state of your data set)
What is Data Governance?
On the other hand, this refers to a set of guidelines, regulations, and rules that govern the administration of the data that is included within an organisation.
(To put it simply, it is the management oversight of your data)
Data by itself is useless. Understanding DIKW.
Just like noise, data does not have any significance beyond its own existence; it becomes meaningless.
The process of transforming data into meaningful insights is described by the DIKW Model, which can be understood from two different vantage points: the act of acquiring the data and the act of interpreting the data.
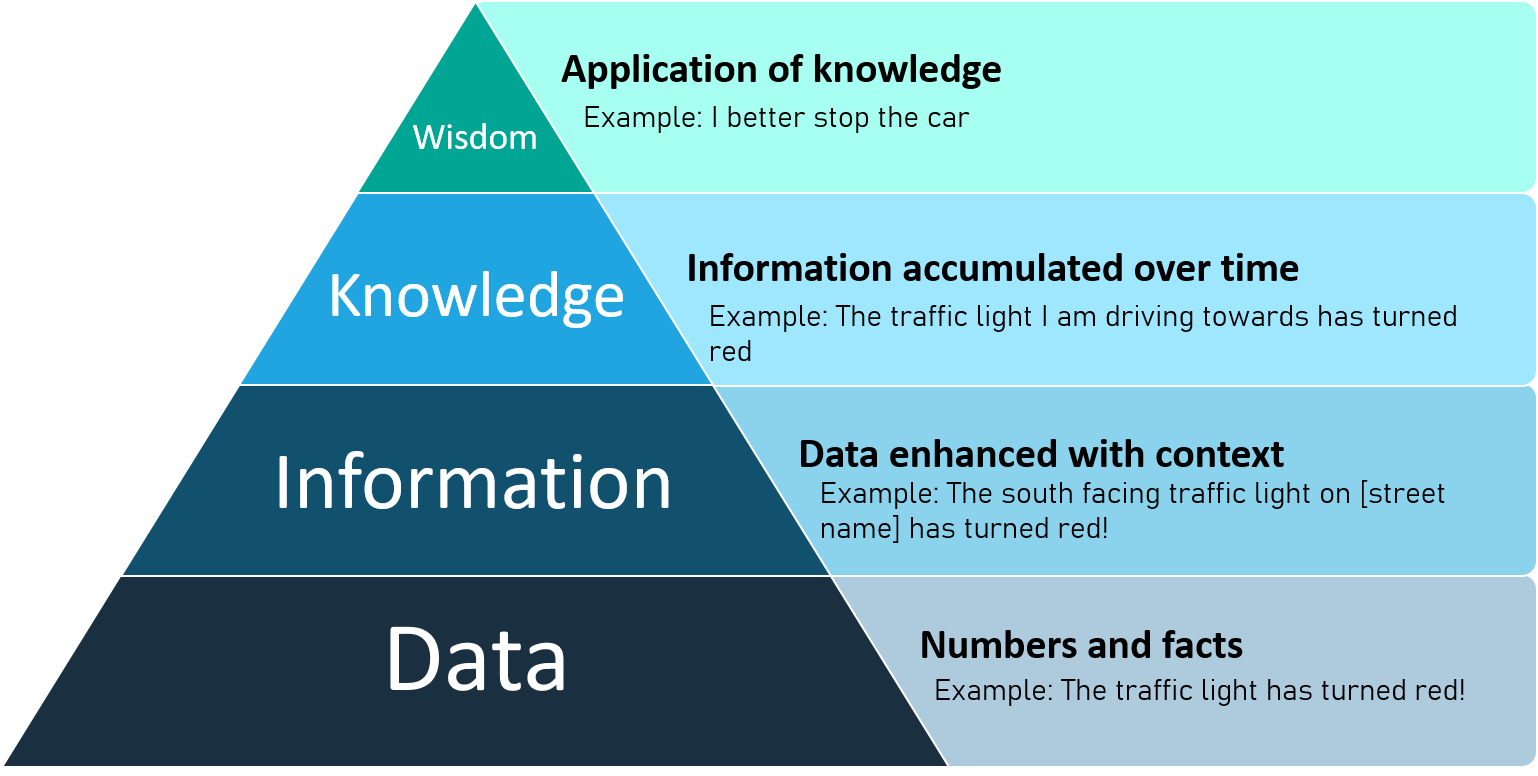
Where does Data Quality & Data Governance overlap?
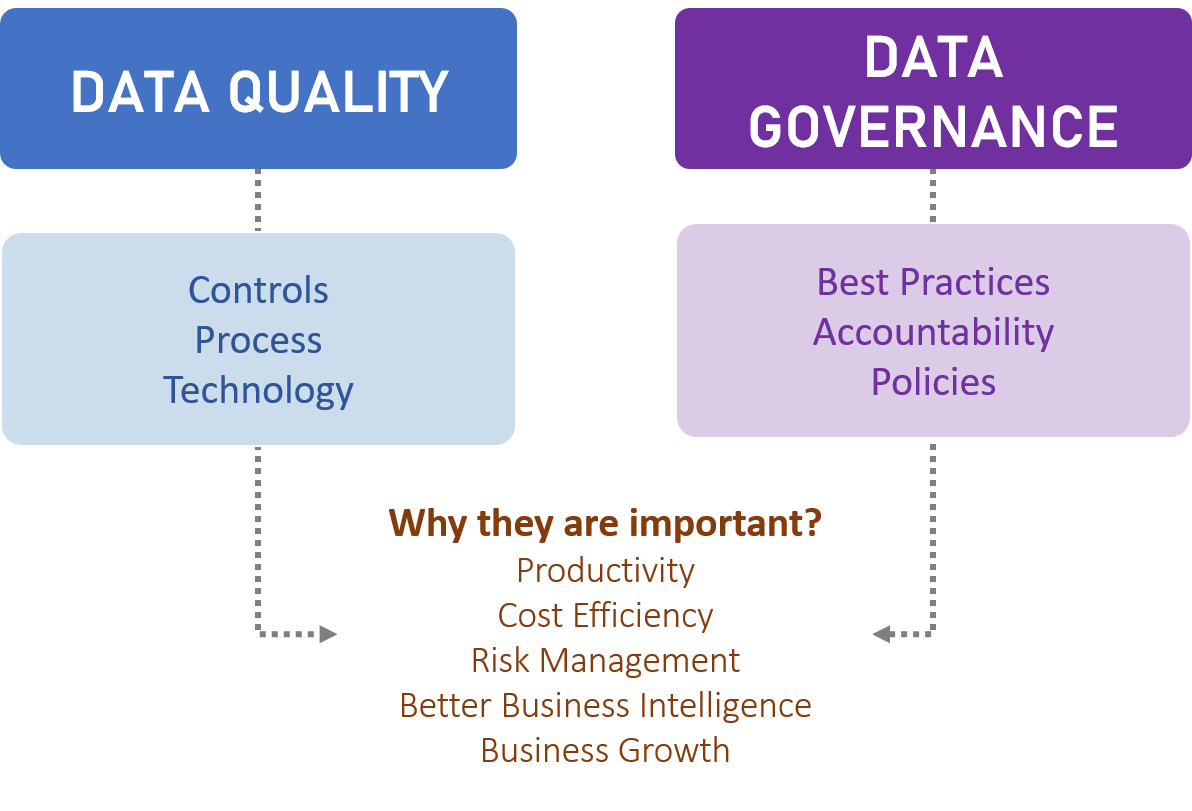
Inter-dependent Relationship
There is a mutually beneficial relationship between data governance and data quality. Organizations that want to derive real value from their data assets need to pay attention to both of these factors since they are inter-dependent.
Neither is a “one over the other” deal, but rather calls for consistent work over time.
Data quality can be thought of as a subset of data governance. Ultimately, it’s difficult to picture efficient data governance without attending to data quality.
In fact, the opposite is also correct. Without a strong governance system in place, it is difficult to attain practically useful standards of data quality.
Problems often emerge far away from the cause
Engaging in data quality and data governance as a continual discipline is tough.
It is one reason why many companies find it challenging and reluctant to engage in the tedious act of data clean-up and constant monitoring of the quality of data for the organization.
Why is it hard?
- Lack of business leadership and commitment
- Failure to link Data Quality and Data Governance to organizational goals and benefits
- Focusing on irrelevant data. By the rule of thumb, the more the data is shared across and beyond the organization, the more important quality and governance becomes.
- Emphasizing method over information. As a result of the constant pressure to meet deadlines, the quality of data and control is often sacrificed.
- Focusing too much on the tools rather than the processes
- Not assigning roles, responsibilities and accountability to people actually responsible for data
- Failing to model the data storage strategy to ensure optimal performance, widespread applicability, and security
What is the implication of inaction then?
- Bad data costs money. Poor data quality has impact on revenue, customer experience & poor decision making
- Ignorance is dangerous. When it comes to dealing with data across multiple systems and organizations, you put yourself in a precarious position if you are unclear of the facts.
- Competitive advantage decline. Your Company’s data loss provides other companies with the opportunity to fill the void that you have failed to solve.
How we have helped our Clients?
Throughout the years that we have spent collaborating with our Clients, we have helped leverage the DIWK methodology to understand not only the data, but also to deliver viable, cost-efficient and lasting business capabilities by understanding the information at hand.
Data Driven Business Success
DATA
Ensuring the uniformity of data collected from various touch points are subjected to strict controls on quality, formats and standardization.
Our Implementations:
Data Quality & Standardization
Workflow Automation
Automated Decisioning
Data Policies on Organization Common Data Formats & Identifiers
Straight-Through Architecture
360-Degree Single View
INFORMATION
Storing all of an organization’s data in one place for easy access and more thorough business intelligence analysis.
Our Implementations:
Master Database References
Security Management & Access Controls
Data Privacy & Sgregation of Data Across Organization & Ranks
KNOWLEDGE
Business Intelligence analytics for improved knowledge of client requirements, safer operations, and more thorough reporting for upper management.
Our Implementations:
Business Intelligence Tools
Real-Time Analytics
Machine Learning
Artificial Intelligence
Self-Service Business Intelligence
WISDOM
Create and provide brand-new products and services in response to market demand by understanding and anticipating the wants and needs of both consumers and own business.
Our Implementations:
Self-Service Customer
On-boarding
Self-Service Transactions Support Portals
Risk Control Self-Assessments
Curating Personalized Contents for Customers
Customer Experience Tools
Contact us today and explore with us how we can help your organization